การสร้างภาพใบหน้าคนร้ายจากคำบรรยายรูปพรรณสัณฐาน
คำสำคัญ:
การเรียนรู้เชิงลึก, การสร้างภาพใบหน้าจากข้อความ, StyleGAN3, Contrastive Language-Image Pre-trainingบทคัดย่อ
ความเป็นมาและวัตถุประสงค์: การแปลงข้อความเป็นรูปภาพได้รับความนิยมแพร่หลายขึ้น เนื่องจากสามารถสร้างประโยชน์ให้กับหลายวงการ เช่น ในด้านการสืบสวนสอบสวนที่ต้องการร่างภาพคนร้าย หรือแม้กระทั่งการตามหาบุคคลสาบสูญ อย่างไรก็ตาม งานวิจัยด้านการแปลงข้อความเป็นรูปภาพส่วนใหญ่มักเป็นการศึกษาบนวัตถุที่ไม่ซับซ้อน เช่น วัตถุทั่วไป ดอกไม้ หรือนก แต่การศึกษาบนใบหน้าคนยังมีไม่มากนัก ส่วนหนึ่งเป็นเพราะฐานข้อมูลใบหน้าคนยังไม่สมบูรณ์ เช่น ฐานข้อมูลของ Labeled Faces in the Wild และ MegaFace ที่มีเพียงรูปภาพแต่ไม่มีคำบรรยาย งานวิจัยนี้เป็นการพัฒนาระบบร่างภาพใบหน้าคนร้ายอัตโนมัติ โดยอาศัยเพียงอินพุตข้อความที่เป็นคำบรรยายลักษณะรูปพรรณสัณฐาน
วิธีดำเนินการวิจัย: ในงานวิจัยนี้ ผู้วิจัยใช้ฐานข้อมูลของ CelebA HQ ซึ่งเป็นฐานข้อมูลที่มีทั้งรูปภาพและคำบรรยาย จากนั้นใช้วิธีเข้ารหัสข้อความด้วย BERT Sentence Encoder และ CLIP Text Encoder เพื่อถอดรหัสคำบรรยายออกเป็นคำศัพท์และแปลงเป็นตัวเลข แล้วนำตัวเลขดังกล่าวไปทดสอบในแบบจำลอง 4 แบบ ได้แก่ StyleGAN3+CLIP, StyleGAN3+CLIP (Fine-Tuning), DC+CLIP (Fine-Tuning) และ DF+CLIP (Fine-Tuning)
ผลการวิจัย: จากผลการทดลองเปรียบเทียบแบบจำลองทั้ง 4 แบบด้วยตัวชี้วัดเชิงปริมาณอย่าง FID พบว่า แบบจำลองที่ใช้ StyleGAN3 เป็น Generator มีประสิทธิภาพสูงสุดในการสร้างภาพที่มีคุณภาพดีและตรงตามคำบรรยาย ทั้งนี้ ผลลัพธ์ที่ดีที่สุดคือเมื่อใช้ StyleGAN3 คู่กันกับแบบจำลอง CLIP ที่ผ่านการ Fine-tuning เพิ่มเติมด้วยชุดข้อมูล CelebA HQ
สรุป: เพื่ออำนวยความสะดวกและเพิ่มประสิทธิภาพของกระบวนการร่างภาพใบหน้าคนร้ายในงานสืบสวนสอบสวน งานวิจัยนี้ทำการพัฒนาระบบร่างภาพใบหน้าคนร้ายโดยอาศัยอินพุตข้อความที่เป็นคำบรรยายรูปพรรณสัณฐานของคนร้ายแต่เพียงอย่างเดียว จากการทดลองผสมผสาน Generative Adversarial Network (GAN) เข้ากับแบบจำลอง CLIP พบว่า การผสมผสาน StyleGAN3 และ CLIP ให้ผลลัพธ์การสร้างภาพใบหน้าได้มีคุณภาพดีและตรงตามคำบรรยายมากที่สุด
การนำไปใช้ประโยชน์ในเชิงปฏิบัติ: งานวิจัยนี้สามารถนำไปพัฒนาต่อยอดเพื่อสร้างระบบร่างภาพใบหน้าคนร้ายจากคำบรรยายรูปพรรณสัณฐานสำหรับช่วยเหลือในการสืบสวนสอบสวน หรือใช้ในบริบทอื่นที่คล้ายกัน เช่น การออกแบบใบหน้าของนางแบบหรือนายแบบใหม่เพื่อประโยชน์ในการสร้างสื่อโฆษณา
References
Jalan, H.J., Maurya, G., Corda, C., Dsouza, S. and Panchal, D., 2020, “Suspect Face Generation,” Proceedings of the 2020 3rd International Conference on Communication System, Computing and IT Applications (CSCITA), 3-4 April 2020, Mumbai, India, pp. 73-78.
Kotian, C., Lokhande, S., Jain, M. and Pavate, A., 2020, “D2F: Description to Face Synthesis Using GAN,” Proceedings of the International Conference on Recent Advances in Computational Techniques (IC-RACT), 27-28 March 2020, Mumbai, India, 8 p.
Nair, K.R., Sam, S.S., Praveena, K.P., Juju, K. and Cherian, S., 2021, “Transfer Learning with Deep Convolutional Neural Networks in Forensic Face Sketch Recognition,” Proceedings of the International Conference on IoT Based Control Networks & Intelligent Systems (ICICNIS 2021), 28-29 June 2021, Kerala, India, pp. 1-5.
Xu, J., Xue, X., Wu, Y. and Mao, X., 2020, “Matching a Composite Sketch to a Photographed Face using Fused HOG and Deep Feature Models,” The Visual Computer, 37 (4), pp. 765-776.
Wadhawan, R., Drall, T., Singh, S. and Chakraverty, S., 2020, “Multi-Attributed and Structured Text-to-Face Synthesis,” IEEE International Conference on Technology, Engineering, Management for Societal impact using Marketing, Entrepreneurship and Talent (TEMSMET), 10-10 December 2020, Bengaluru, India, pp. 1-7.
Sun, J., Li, Q., Wang, W., Zhao, J. and Sun, Z., 2021, “Multi-caption Text-to-Face Synthesis: Dataset and Algorithm,” Proceedings of the 29th ACM International Conference on Multimedia, Association for Computing Machinery, 20-24 October 2021, New York, USA, pp. 2290–2298.
Oza, M., Chanda, S. and Doerman, D., 2022, “Semantic Text-to-Face GAN-ST2F,” arXiv preprint arXiv:2107.10756. https://doi.org/10.48550/arXiv.2107.10756
Wang, T., Zhang, T. and Lovell, B., 2021, “Faces la Carte: Text-to-Face Generation via Attribute Disentanglement,” Winter Conference of Applications on Computer Vision (WACV), 5-9 January 2021, Virtually, pp. 3380-3388.
Sun, J., Deng, Q., Li, Q., Sun, M., Ren, M. and Sun, Z., 2022, “AnyFace: Free-style Text-to-Face Synthesis and Manipulation,” arXiv preprint arXiv:2203.15334v1. https://doi.org/10.48550/arXiv.2203.15334
Li, Z., Min, M.R., Li, K. and Xu, C., 2022, “StyleT2I: Toward Compositional and High-Fidelity Text-to-Image Synthesis,” Conference on Computer Vision and Pattern Recognition (CVPR), 19-24 June 2022, New Orleans, Louisiana, USA, pp. 18197-18207.
Hermosilla, G., Tapia, D.H., Allende-Cid, H., Castro, G.F. and Vera, E., 2021, “Thermal Face Generation Using StyleGAN,” IEEE Access, 9, pp. 80511-80523.
Tao, M., Tang, H., Wu, F., Jing, X., Bao, B. and Xu, C., 2022, “DF-GAN: A Simple and Effective Baseline for Text-to-Image Synthesis,” Conference on Computer Vision and Pattern Recognition (CVPR), 19-24 June 2022, New Orleans, Louisiana, USA, pp. 16515-16525.
Patashnik, O., Wu, Z., Shechtman, E., Cohen-Or, D. and Lischinski, D., 2021, “StyleCLIP: Text-Driven Manipulation of StyleGAN Imagery,” Proceedings of the International Conference on Computer Vision (ICCV), Virtually, 11-17 October 2021, Virtually, pp. 2085-2094.
Deorukhkar, K., Kadamala, K. and Menezes, E., 2022, “FGTD: Face Generation from Textual Description,” Inventive Communication and Computational Technologies, Lecture Notes in Networks and Systems, 311, pp. 547-562.
Karras, T., Aittala, M., Laine, S., Härkönen, E., Hellsten, J., Lehtinen, J. and Aila, T., 2021, “Alias-Free Generative Adversarial Networks,” Conference on Neural Information Processing Systems (NeurIPS 2021), 6-14 December 2021, Virtually, 12 p.
Radford, A., Kim, J.W., Hallacy, C., Ramesh, A., Goh, G., Agarwal, S., Sastry, G., Askell, A., Mishkin, P., Clark, J., Krueger, G. and Sutskever, I., 2021, “Learning Transferable Visual Models From Natural Language Supervision,” Proceedings of the 38th International Conference on Machine Learning (PMLR), 18-24 July 2021, Virtually, 16 p.
Devlin, J., Chang, M., Lee, K. and Toutanova, K., 2019, “BERT: Pre-training of Deep Bidirectional Transformers for Language Understanding,” Conference of the North American Chapter of the Association for Computational Linguistics: Human Language Technologies (NAACL), 2-7 June 2019, Minneapolis, Minnesota, USA, pp. 4171-4186.
Cui, J., Jin, L., Kuang, H., Xu, Q. and Schwertfeger, S., 2021, “Underwater Depth Estimation for Spherical Images,” Journal of Robotics, 2021, 12 p.
Heusel, M., Ramsauer, H., Unterthiner, T., Nessler, B. and Hochreiter, S., 2017, “GANs Trained by a Two Time-scale Update Rule Converge to a Local Nash Equilibrium,” Proceedings of the 31st International Conference on Neural Information Processing Systems (NIPS'17), 4-9 December 2017, Long Beach, CA, USA, pp. 6629–6640.
Radford, A., Metz, L. and Chintala, S., 2016, “Unsupervised Representation Learning with Deep Convolutional Generative Adversarial Networks,” International Conference on Learning Representations (ICLR), 2-4 May 2016, San Juan, Puerto Rico.
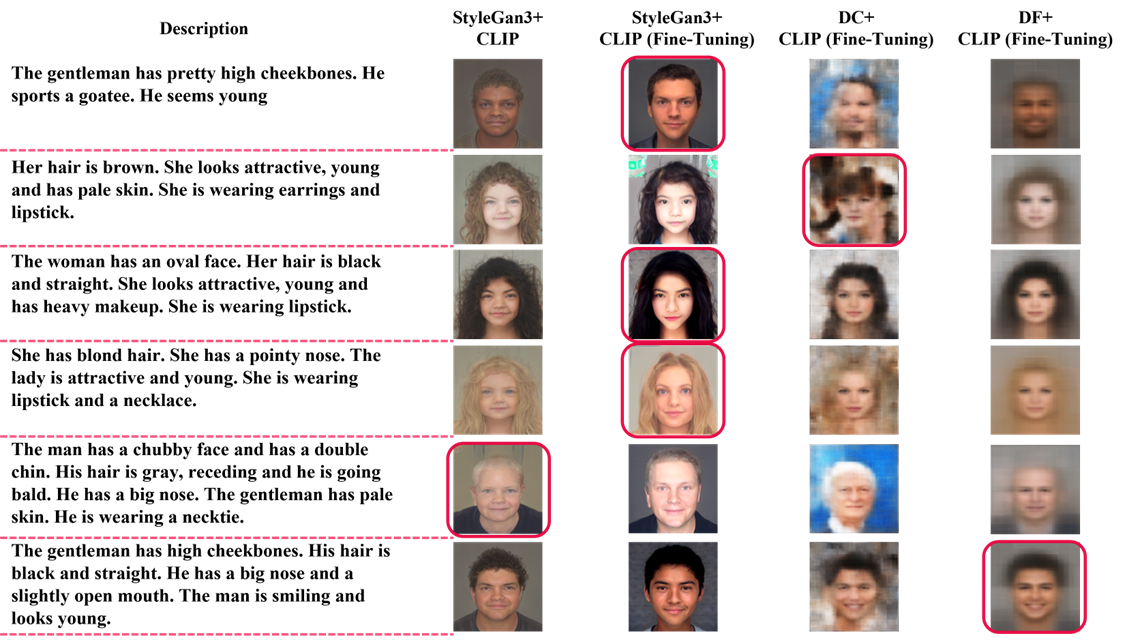
Downloads
เผยแพร่แล้ว
How to Cite
ฉบับ
บท
License
Copyright (c) 2024 มหาวิทยาลัยเทคโนโลยีพระจอมเกล้าธนบุรี

This work is licensed under a Creative Commons Attribution-NonCommercial-NoDerivatives 4.0 International License.
เนื้อหาของบทความที่ตีพิมพ์ในวารสาร Science and Engineering Connect ในทุกรูปแบบ รวมถึงข้อความ สมการ สูตร ตาราง ภาพ ตลอดจนภาพประกอบในรูปแบบอื่นใด เป็นลิขสิทธิ์ของมหาวิทยาลัยเทคโนโลยีพระจอมเกล้าธนบุรี การนำเนื้อหา ไม่ว่าจะในรูปแบบใด ของบทความไปใช้ประโยชน์ในเชิงพาณิชย์ ต้องได้รับอนุญาตจากบรรณาธิการวารสารอย่างเป็นลายลักษณ์อักษรก่อนเท่านั้น