Future Growing Seasons: Bias Correction with SVR and QDM for Indonesian Temperature Projection under RCP 2.6 and RCP 8.5
DOI:
https://doi.org/10.59796/jcst.V15N2.2025.100Keywords:
bias correction, SVR, QDM, mean temperature, GSL, RCP 2.6, RCP 8.5Abstract
Human activities have significantly contributed to greenhouse gas (GHG) emissions, escalating global temperatures and exacerbating extreme weather events, which pose serious agricultural threats by disrupting crop growth. Climate researchers employ Representative Concentration Pathways (RCPs) to forecast future GHG scenarios. Downscaling techniques have improved predictions of Growing Season Lengths (GSL) predictions and mean temperatures (Tmean), both of which are crucial for agricultural planning. This study evaluated Support Vector Regression (SVR) and Quantile Delta Mapping (QDM) for projecting Tmean and its impact on GSL under RCP 2.6 and RCP 8.5. Bias correction was applied to historical Tmean data using both methods, based on ERA5 data. SVR showed a lower Root Mean Square Error (RMSE) (0.6 vs 1.1) and a slightly higher correlation (0.6 vs. 0.5) than QDM. However, QDM was chosen for Tmean projection due to its superior data homogeneity and better alignment of standard deviation with observed values. Projections indicated a significant Tmean increase after 2026 under both RCPs, with Tmean under RCP 8.5 expected to exceed 30°C between 2050 and 2100, necessitating heat-resistant crop varieties. Greater increases in GSL under RCP 2.6 underscored the need for effective mitigation strategies. This study emphasizes adaptive farming practices and recommends integrating quantile-based and machine learning methods into future climate projections to enhance agricultural resilience.
References
Amien, L. I., & Runtunuwu, E. (2009). Agrometeorological Data And Rainfall Forecasting For Crop Simulation. Jurnal Sumber Daya Lahan, 3(2), 61–72. https://doi.org/10.2018/jsdl.v3i02.185
Arslantaş, E. E., & Yeşilırmak, E. (2020). Changes in the climatic growing season in Western Anatolia, Turkey. Meteorological Applications, 27(2), Article e1897. https://doi.org/10.1002/met.1897
Largeau, A., Wongprathed, J., Wongwailikhit, K., Dietrich, N., Soottitantawat, A., Methaapanon, R., Beghin, R., Frayssignes, J., & Hébrard, G. (2024). An inspiring way to reach carbon neutrality: Aqueous carbonation of coal and biomass ashes. Paper presented at the International Congress of Chemical and Process Engineering, Prague, Czech Republic. https://hal.archives-ouvertes.fr/hal-04782905
Barnard, D. M., Knowles, J. F., Barnard, H. R., Goulden, M. L., Hu, J., Litvak, M. E., & Molotch, N. P. (2018). Reevaluating growing season length controls on net ecosystem production in evergreen conifer forests. Scientific Reports, 8(1), Article 36065. https://doi.org/10.1038/s41598-018-36065-0
Brown, M. E., Antle, J. M., Backlund, P., Carr, E. R., Easterling, W. E., Walsh, M. K., … & Tebaldi, C. (2015). Climate Change, Global Food Security, and the U.S. Food System. Retrieved from https://ageconsearch.umn.edu/record/337546/?v=pdf
Calinger, K., & Curtis, P. (2023). A century of climate warming results in growing season extension: Delayed autumn leaf phenology in north-central North America. PLOS ONE, 18(3), Article e0282391. https://doi.org/10.1371/journal.pone.0282391
Cannon, A. J., Sobie, S. R., & Murdock, T. Q. (2015). Bias correction of GCM precipitation by quantile mapping: How well do methods preserve changes in quantiles and extremes?. Journal of Climate, 28(17), 6938–6959. https://doi.org/10.1175/JCLI-D-14-00754.1
Cech, R., Leisch, F., & Zaller, J. G. (2022). Pesticide use and associated greenhouse gas emissions in sugar beet, apples, and viticulture in Austria from 2000 to 2019. Agriculture, 12(6), Article 879. https://doi.org/10.3390/agriculture12060879
Chancellor, W., Hughes, N., Zhao, S., Soh, W. Y., Valle, H., & Boult, C. (2021). Controlling for the effects of climate on total factor productivity: A case study of Australian farms. Food Policy, 102, Article 102091. https://doi.org/10.1016/j.foodpol.2021.102091
Chen, W., Zorn, P., White, L., Olthof, I., Zhang, Y., Fraser, R., & Leblanc, S. (2016). Decoupling between Plant Productivity and Growing Season Length under a Warming Climate in Canada’s Arctic. American Journal of Climate Change, 5(3), 334–359. https://doi.org/10.4236/ajcc.2016.53026
Cho, D., Yoo, C., Im, J., & Cha, D. H. (2020). Comparative assessment of various machine learning-based bias correction methods for numerical weather prediction model forecasts of extreme air temperatures in urban areas. Earth and Space Science, 7(4), Article e2019EA000740. https://doi.org/10.1029/2019EA000740
Fauzi, F., Kuswanto, H., & Atok, R. M. (2020). Bias correction and statistical downscaling of earth system models using quantile delta mapping (QDM) and bias correction constructed analogues with quantile mapping reordering (BCCAQ). Journal of Physics: Conference Series, 1538(1), 1–9. https://doi.org/10.1088/1742-6596/1538/1/012050
Fernie, E., Tan, D. K. Y., Liu, S. Y., Ullah, N., & Khoddami, A. (2022). Post-Anthesis Heat Influences Grain Yield, Physical and Nutritional Quality in Wheat: A Review. Agriculture (Switzerland), 12(6), 1–24. https://doi.org/10.3390/agriculture12060886
Gouveia, C. M., Justino, F., Gurjao, C., Zita, L., & Alonso, C. (2023). Revisiting Climate-Related Agricultural Losses across South America and Their Future Perspectives. Atmosphere, 14(8), 1–20. https://doi.org/10.3390/atmos14081303
Hinze, J., Albrecht, A., & Michiels, H. G. (2023). Climate-Adapted Potential Vegetation—A European Multiclass Model Estimating the Future Potential of Natural Vegetation. Forests, 14(2), 1–19. https://doi.org/10.3390/f14020239
Huang, L., Fang, X., Zhang, T., Wang, H., Cui, L., & Liu, L. (2023). Evaluation of surface temperature and pressure derived from MERRA-2 and ERA5 reanalysis datasets and their applications in hourly GNSS precipitable water vapor retrieval over China. Geodesy and Geodynamics, 14(2), 111–120. https://doi.org/10.1016/j.geog.2022.08.006
Hudson, A. R., Smith, W. K., Moore, D. J. P., & Trouet, V. (2022). Length of growing season is modulated by Northern Hemisphere jet stream variability. International Journal of Climatology, 42(11), 5644–5660. https://doi.org/10.1002/joc.7553
Hunter, R. D., & Meentemeyer, R. K. (2005). Climatologically aided mapping of daily precipitation and temperature. Journal of Applied Meteorology, 44(10), 1501–1510. https://doi.org/10.1175/JAM2295.1
Intergovernmental Panel on Climate Change (IPCC). (2015). Climate change 2014: Synthesis report. Contribution of Working Groups I, II and III to the Fifth Assessment Report of the Intergovernmental Panel on Climate Change (R. K. Pachauri & L. A. Meyer, Eds.). In Managing the Risks of Extreme Events and Disasters to Advance Climate Change Adaptation: Special Report of the Intergovernmental Panel on Climate Change. IPCC. https://doi.org/10.1017/CBO9781139177245.003
Jubb, I., Canadell, P., & Dix, M. (2013). Representative concentration pathways. Australian Government, Department of the Environment. https://www.environment.gov.au/
Khairulbahri, M. . (2022). Understanding the Impacts of Climate Change on Rice Production: A Qualitative Perspective. Science & Technology Asia, 27(1), 169–179. https://ph02.tci-thaijo.org/index.php/SciTechAsia/article/view/242411
Kumar, V., Kumari, A., Price, A. J., Bana, R. S., Singh, V., & Bamboriya, S. D. (2023). Impact of futuristic climate variables on weed biology and herbicidal efficacy: A review. Agronomy, 13(2), Article 559. https://doi.org/10.3390/agronomy13020559
Kumari, A., Lakshmi, G. A., Krishna, G. K., Patni, B., Prakash, S., Bhattacharyya, M., & Verma, K. K. (2022). Climate change and its impact on crops: A comprehensive investigation for sustainable agriculture. Agronomy, 12(12), Article 3008. https://doi.org/10.3390/agronomy12123008
Kuswanto, H., Kravitz, B., Miftahurrohmah, B., Fauzi, F., Sopahaluwaken, A., & Moore, J. (2022). Impact of solar geoengineering on temperatures over the Indonesian Maritime Continent. International Journal of Climatology, 42(5), 2795–2814. https://doi.org/10.1002/joc.7391
Liu, E., Zhou, G., He, Q., Wu, B., Zhou, H., & Gu, W. (2022). Climatic mechanism of delaying the start and advancing the end of the growing season of Stipa krylovii in a semi-arid region from 1985 to 2018. Agronomy, 12(8), Article 1906. https://doi.org/10.3390/agronomy12081906
Miftahurrohmah, B., Kuswanto, H., Pambudi, D. S., Fauzi, F., & Atmaja, F. (2024). Assessment Assessment of of the the Support Support Vector Regression Regression and Random Random Forest Algorithms in the Bias Bias Correction Process on Temperatures Algorithms. Procedia Computer Science, 234, 637–644. https://doi.org/10.1016/j.procs.2024.03.049
Montero-Martínez, M. J., & Andrade-Velázquez, M. (2022). Effects of urbanization on extreme climate indices in the Valley of Mexico Basin. Atmosphere, 13(5), Article 785. https://doi.org/10.3390/atmos13050785
National Climate Change Adaptation Research Facility. (2017). How to use climate change scenarios. Retrieved from https://coastadapt.com.au/how-to-pages/how-to-use-climate-change-scenarios-to-evaluate-risk-plan-and-make-decisions
Ngongondo, C. O. S. M. O., Tallaksen, L. M., & Xu, C. Y. (2014). Growing season length and rainfall extremes analysis in Malawi. In Hydrology in a changing world: Environmental and human dimensions (pp. 361–365). International Association of Hydrological Sciences (IAHS) Publications, 363, 361-365.
Park, T., Ganguly, S., Tømmervik, H., Euskirchen, E. S., Høgda, K. A., Karlsen, S. R., & Myneni, R. B. (2016). Changes in growing season duration and productivity of northern vegetation inferred from long-term remote sensing data. Environmental Research Letters, 11(8), Article 084001. https://doi.org/10.1088/1748-9326/11/8/084001
Petchchedchoo, P., Petcharaks, N., Piratrakul, P., & Wongmuek, K. (2024). Analysis of the carbon footprint of academic gowns: a case study of thai university. Journal of Current Science and Technology,14(2), Article 46. https://doi.org/10.59796/jcst.V14N2.2024.46
Potisomporn, P., Adcock, T. A. A., & Vogel, C. R. (2023). Evaluating ERA5 reanalysis predictions of low wind speed events around the UK. Energy Reports, 10, 4781–4790. https://doi.org/10.1016/j.egyr.2023.11.035
Qi, Y., Zhang, Q., Hu, S., Wang, R., Wang, H., Zhang, K., & Yang, Y. (2022). Effects of high temperature and drought stresses on growth and yield of summer maize during grain filling in North China. Agriculture, 12(11), Article 1948. https://doi.org/10.3390/agriculture12111948
Rajulapati, C. R., & Papalexiou, S. M. (2023). Precipitation bias correction: A novel semi-parametric quantile mapping method. Earth and Space Science, 10(4), Article e2023EA002823. https://doi.org/10.1029/2023EA002823
Seo, G.-Y., & Ahn, J.-B. (2023). Comparison of bias correction methods for summertime daily rainfall in South Korea using quantile mapping and machine learning models. Atmosphere, 14(7), Article 1057. https://doi.org/10.3390/atmos14071057
Tan, M. L., Armanuos, A. M., Ahmadianfar, I., Demir, V., Heddam, S., Al-Areeq, A. M., & Yaseen, Z. M. (2023). Evaluation of NASA POWER and ERA5-Land for estimating tropical precipitation and temperature extremes. Journal of Hydrology, 624, Article 129940. https://doi.org/10.1016/j.jhydrol.2023.129940
Tan, Y., Guzman, S. M., Dong, Z., & Tan, L. (2020). Selection of effective GCM bias correction methods and evaluation of hydrological response under future climate scenarios. Climate, 8(10), Article 108. https://doi.org/10.3390/cli8100108
Ukaew, S., Tungtakanpoung, D., & Chongsithiphol, S. (2020). An assessment of life cycle greenhouse gas emissions for day spa services in Eastern Thailand: A case study in Chonburi, Rayong, and Trad provinces. Naresuan University Journal: Science and Technology, 28(1), Article 1. https://doi.org/10.14456/nujst.2020.1
Villa-Falfán, C., Valdés-Rodríguez, O. A., Vázquez-Aguirre, J. L., & Salas-Martínez, F. (2023). Climate indices and their impact on maize yield in Veracruz, Mexico. Atmosphere, 14(5), Article 778. https://doi.org/10.3390/atmos14050778
Wang, F., & Tian, D. (2022). On deep learning-based bias correction and downscaling of multiple climate models simulations. Climate Dynamics, 59(11–12), 3451–3468. https://doi.org/10.1007/s00382-022-06277-2
Wang, F., Tian, D., & Carroll, M. (2023). Customized deep learning for precipitation bias correction and downscaling. Geoscientific Model Development, 16(2), 535–556. https://doi.org/10.5194/gmd-16-535-2023
Wang, J. W., Li, M., Zhang, G. Y., Zhang, H. R., & Yu, C. Q. (2020). Growing season precipitation rather than growing season length predominates maximum normalized difference vegetation index in alpine grasslands on the Tibetan Plateau. Sustainability, 12(3), Article 968. https://doi.org/10.3390/su12030968
Xavier, A. C. F., Martins, L. L., Rudke, A. P., de Morais, M. V. B., Martins, J. A., & Blain, G. C. (2022). Evaluation of Quantile Delta Mapping as a bias‐correction method in maximum rainfall dataset from downscaled models in São Paulo state (Brazil). International Journal of Climatology, 42(1), 175–190. https://doi.org/10.1002/joc.7238
Yilmaz, M. (2023). Accuracy assessment of temperature trends from ERA5 and ERA5-Land. The Science of The Total Environment, 856(2), Article 159182. https://doi.org/10.1016/j.scitotenv.2022.159182
Zare, M., Azam, S., Sauchyn, D., & Basu, S. (2023). Assessment of meteorological and agricultural drought indices under climate change scenarios in the South Saskatchewan River Basin, Canada. Sustainability, 15(7), Article 5907. https://doi.org/10.3390/su15075907
Zhou, Y. (2020). Relative contribution of growing season length and amplitude to long-term trend and interannual variability of vegetation productivity over northeast China. Forests, 11(1), Article 112. https://doi.org/10.3390/f11010112
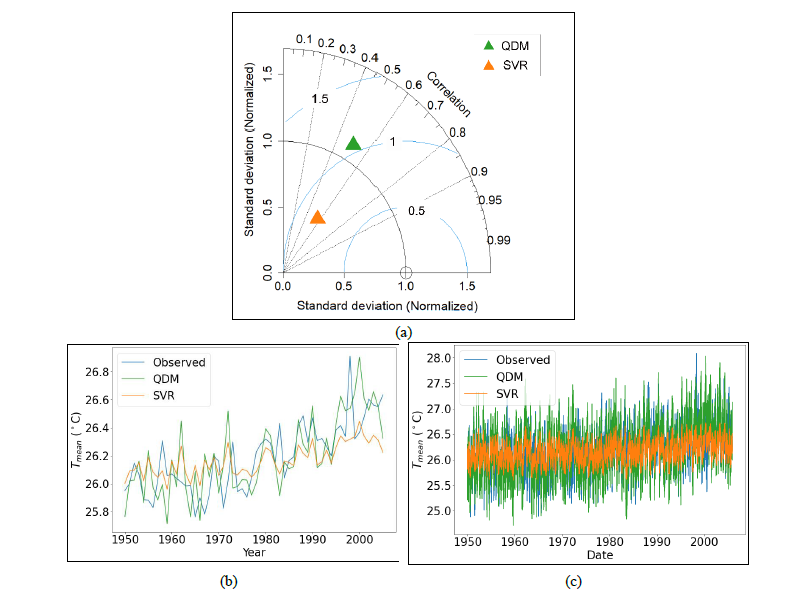
Downloads
Published
How to Cite
Issue
Section
Categories
License
Copyright (c) 2025 Journal of Current Science and Technology

This work is licensed under a Creative Commons Attribution-NonCommercial-NoDerivatives 4.0 International License.