Artificial Intelligence, Cybersight Detection of Diabetic Retinopathy in the Elderly in Vietnam
DOI:
https://doi.org/10.59796/jcst.V15N1.2025.80Keywords:
Cybersight, artificial intelligence, diabetes, diabetic retinopathyAbstract
Diabetic retinopathy (DR) is a highly prevalent cause of vision loss worldwide. Detection of DR requires substantial human resources and high medical costs. Therefore, the use of diagnostic software has been recently explored. The study aimed to assess the results of DR diagnoses by Cybersight, an artificial intelligence software. A total of 1,012 patients with type 2 diabetes mellitus (1,943 eyes) with a mean age of 74.61 ± 6.73 years were included. Comprehensive demographic and clinical data were gathered, and all patients underwent color fundus photography following Cybersight's standardized protocols. The study compared Cybersight's accuracy with that of ophthalmologists in identifying key DR lesions, including retinal microvascular changes, exudates, hemorrhages, the diagnosis and staging of DR, using sensitivity, specificity, and weighted Kappa metrics. The prevalence of DR was 16.2%. A high level of agreement was found between Cybersight and ophthalmologists in DR diagnosis, with a sensitivity of 85.0%, specificity of 95.8%, and a weighted Kappa of 0.78. The presence of cataracts and the degree of pupil dilation notably impacted on the accuracy of DR diagnosis. The results have important implications for the potential application of Cybersight as a low-cost and effective tool for diabetic eye screening.
References
Abràmoff, M. D., Lavin, P. T., Birch, M., Shah, N., & Folk, J. C. (2018). Pivotal trial of an autonomous AI-based diagnostic system for detection of diabetic retinopathy in primary care offices. npj Digital Medicine, 1(1), Article 39. https://doi.org/10.1038/s41746-018-0040-6
Ausawalaithong, W., Thirach, A., Marukatat, S., & Wilaiprasitporn, T. (2018, November 21-24). Automatic lung cancer prediction from chest X-ray images using the deep learning approach [Conference presentation]. 2018 11th biomedical engineering international conference (BMEiCON), Chiang Mai, Thailand.
American Diabetes Association. (2022). Standards of medical care in diabetes-2022 abridged for primary care providers. Clinical Diabetes, 40(1), 10-38. https://doi.org/10.2337/cd22-as01
Bellemo, V., Lim, Z. W., Lim, G., Nguyen, Q. D., Xie, Y., Yip, M. Y., ... & Ting, D. S. (2019). Artificial intelligence using deep learning to screen for referable and vision-threatening diabetic retinopathy in Africa: a clinical validation study. The Lancet Digital Health, 1(1), e35-e44. https://doi.org/10.1016/s2589-7500(19)30004-4
Bhaskaranand, M., Ramachandra, C., Bhat, S., Cuadros, J., Nittala, M. G., Sadda, S. R., & Solanki, K. (2019). The Value of Automated Diabetic Retinopathy Screening with the EyeArt System: A Study of More Than 100,000 Consecutive Encounters from People with Diabetes. Diabetes Technology & Therapeutics, 21(11), 635-643. https://doi.org/10.1089/dia.2019.0164
Cao, S., Zhang, R., Jiang, A., Kuerban, M., Wumaier, A., Wu, J., ... & Chen, R. (2023). Application effect of an artificial intelligence-based fundus screening system: evaluation in a clinical setting and population screening. Biomedical Engineering Online, 22(1), Article 38. https://doi.org/10.1186/s12938-023-01097-9
Cohen, J. (1968). Weighted kappa: nominal scale agreement with provision for scaled disagreement or partial credit. Psychological Bulletin, 70(4), 213-220. https://doi.org/10.1037/h0026256
Cole, E., Valikodath, N. G., Al-Khaled, T., Bajimaya, S., Sagun, K. C., Chuluunbat, T., ... & Chan, R. P. (2022). Evaluation of an Artificial Intelligence System for Retinopathy of Prematurity Screening in Nepal and Mongolia. Ophthalmology Science, 2(4), Article 100165. https://doi.org/10.1016/j.xops.2022.100165
Dimore, A. L., Edosa, Z. K., & Mitiku, A. A. (2023). Glycemic control and diabetes complications among adult type 2 diabetic patients at public hospitals in Hadiya zone, Southern Ethiopia. PLoS One, 18(3), Article e0282962. https://doi.org/10.1371/journal.pone.0282962
Farmaki, P., Damaskos, C., Garmpis, N., Garmpi, A., Savvanis, S., & Diamantis, E. (2020). Complications of the Type 2 Diabetes Mellitus. Current Cardiology Reviews, 16(4), 249-251. https://doi.org/10.2174/1573403x1604201229115531
Feldman, B. H., Aggarwal, S., Reddy, V., Thomas, M., & Harvey, M. D. (2024). Pupil Measurements prior to Refractive Surgery. Retrieved from https://eyewiki.org/Pupil_Measurements_prior_to_Refractive_Surgery
Gilbert, M. J., & Sun, J. K. (2020). Artificial Intelligence in the assessment of diabetic retinopathy from fundus photographs. Seminars in Ophthalmology, 35(7-8), 325-332. https://doi.org/10.1080/08820538.2020.1855358
Gu, C., Wang, Y., Jiang, Y., Xu, F., Wang, S., Liu, R., ... & Chen, J. (2024). Application of artificial intelligence system for screening multiple fundus diseases in Chinese primary healthcare settings: a real-world, multicentre and cross-sectional study of 4795 cases. British Journal of Ophthalmology, 108(3), 424-431. https://doi.org/10.1136/bjo-2022-322940
He, J., Cao, T., Xu, F., Wang, S., Tao, H., Wu, T., Sun, L., & Chen, J. (2020). Artificial intelligence-based screening for diabetic retinopathy at community hospital. Eye (Lond), 34(3), 572-576. https://doi.org/10.1038/s41433-019-0562-4
Ipp, E., Liljenquist, D., Bode, B., Shah, V. N., Silverstein, S., Regillo, C. D., ... & EyeArt Study Group. (2021). Pivotal Evaluation of an Artificial Intelligence System for Autonomous Detection of Referrable and Vision-Threatening Diabetic Retinopathy. JAMA Network Open, 4(11), Article e2134254. https://doi.org/10.1001/jamanetworkopen.2021.34254
Klein, R., Klein, B. E., Neider, M. W., Hubbard, L. D., Meuer, S. M., & Brothers, R. J. (1985). Diabetic retinopathy as detected using ophthalmoscopy, a nonmyciriatic camera and a standard fundus camera. Ophthalmology, 92(4), 485-491. https://doi.org/10.1016/S0161-6420(85)34003-4
Lupidi, M., Danieli, L., Fruttini, D., Nicolai, M., Lassandro, N., Chhablani, J., & Mariotti, C. (2023). Artificial intelligence in diabetic retinopathy screening: clinical assessment using handheld fundus camera in a real-life setting. Acta Diabetologica, 60(8), 1083-1088. https://doi.org/10.1007/s00592-023-02104-0
Malerbi, F. K., Andrade, R. E., Morales, P. H., Stuchi, J. A., Lencione, D., de Paulo, J. V., ... & Belfort Jr, R. (2022). Diabetic Retinopathy Screening Using Artificial Intelligence and Handheld Smartphone-Based Retinal Camera. Journal of Diabetes Science and Technology, 16(3), 716-723. https://doi.org/10.1177/1932296820985567
Mathenge, W., Whitestone, N., Nkurikiye, J., Patnaik, J. L., Piyasena, P., Uwaliraye, P., ... & Jaccard, N. (2022). Impact of artificial intelligence assessment of diabetic retinopathy on referral service uptake in a low-resource setting: the RAIDERS randomized trial. Ophthalmology Science, 2(4), Article 100168. https://doi.org/https://doi.org/10.1016/j.xops.2022.100168
Manosroi, W., Phimphilai, M., Waisayanand, N., Buranapin, S., Deerochanawong, C., Gunaparn, S., Phrommintikul, A., & M.D, W. (2023). Glycated hemoglobin variability and the risk of cardiovascular events in patients with prediabetes and type 2 diabetes mellitus: A post-hoc analysis of a prospective and multicenter study. Journal of Diabetes Investigation, 14, 1391-1400. https://doi.org/10.1111/jdi.14073
Ong, K. L., Stafford, L. K., McLaughlin, S. A., Boyko, E. J., Vollset, S. E., Smith, A. E., ... & Brauer, M. (2023). Global, regional, and national burden of diabetes from 1990 to 2021, with projections of prevalence to 2050: a systematic analysis for the Global Burden of Disease Study 2021. The Lancet, 402(10397), 203-234. https://doi.org/10.1016/S0140-6736(23)01301-6
Pechprasarn, S., Wattanapermpool, O., Warunlawan, M., Homsud, P., & Akarajarasroj, T. (2023). Identification of Important Factors in the Diagnosis of Breast Cancer Cells Using Machine Learning Models and Principal Component Analysis. Journal of Current Science and Technology, 13(3), 642-656.
Pechprasarn,S., Suechoey, N., Pholtrakoolwong, N., Tanedvorapinyo, P., & Toboonliang, Y.(2024). Optimizing lung cancer diagnosis with machine learning and feature selection methods. Journal of Current Science and Technology, 14(3), Article 55. https://doi.org/10.59796/jcst.V14N3.2024.55
Phan, D. H., Vu, T. T., Doan, V. T., Le, T. Q., Nguyen, T. D., & Van Hoang, M. (2022). Assessment of the risk factors associated with type 2 diabetes and prediabetes mellitus: A national survey in Vietnam. Medicine (Baltimore), 101(41), Article e31149. https://doi.org/10.1097/md.0000000000031149
Rice, J. (2011). Cataract and diabetic retinopathy. Community Eye Health, 24(75), Article 9.
Ruamviboonsuk, P, Tiwari, R, Sayres, R, Nganthavee, V, Hemarat, K,…..& Webster, D. R. (2022). Real-time diabetic retinopathy screening by deep learning in a multisite national screening programme: a prospective interventional cohort study. The Lancet Digital Health, 4(4), e235-e244. https://doi.org/10.1016/S2589-7500(22)00017-6.
Ruta, L. M., Magliano, D. J., Lemesurier, R., Taylor, H. R., Zimmet, P. Z., & Shaw, J. E. (2013). Prevalence of diabetic retinopathy in Type 2 diabetes in developing and developed countries. Diabetic Medicine, 30(4), 387-398. https://doi.org/10.1111/dme.12119
Srisubat, A., Kittrongsiri, K., Sangroongruangsri, S., Khemvaranan, C., Shreibati, J. B….. & Ruamviboonsuk, P. (2023). Cost-Utility Analysis of Deep Learning and Trained Human Graders for Diabetic Retinopathy Screening in a Nationwide Program. Ophthalmology and Therapy, 12, 1339–1357. https://doi.org/10.1007/s40123-023-00688-y
Scotland, G. S., McNamee, P., Philip, S., Fleming, A. D., Goatman, K. A., Prescott, G. J., ... & Olson, J. A. (2007). Cost-effectiveness of implementing automated grading within the national screening programme for diabetic retinopathy in Scotland. British Journal of Ophthalmology, 91(11), 1518-1523. https://doi.org/10.1136/bjo.2007.120972
Tufail, A., Rudisill, C., Egan, C., Kapetanakis, V. V., Salas-Vega, S., Owen, C. G., ... & Rudnicka, A. R. (2017). Automated Diabetic Retinopathy Image Assessment Software: Diagnostic Accuracy and Cost-Effectiveness Compared with Human Graders. Ophthalmology, 124(3), 343-351. https://doi.org/10.1016/j.ophtha.2016.11.014
Uy, H., Fielding, C., Hohlfeld, A., Ochodo, E., Opare, A., Mukonda, E., ... & Engel, M. E. (2023). Diagnostic test accuracy of artificial intelligence in screening for referable diabetic retinopathy in real-world settings: A systematic review and meta-analysis. PLOS Global Public Health, 3(9), Article e0002160. https://doi.org/10.1371/journal.pgph.0002160
Vought, R., Vought, V., Shah, M., Szirth, B., & Bhagat, N. (2023). EyeArt artificial intelligence analysis of diabetic retinopathy in retinal screening events. International Ophthalmology, 43(12), 4851-4859. https://doi.org/10.1007/s10792-023-02887-9
Whitestone, N., Nkurikiye, J., Patnaik, J. L., Jaccard, N., Lanouette, G., Cherwek, D. H., ... & Mathenge, W. (2024). Feasibility and acceptance of artificial intelligence-based diabetic retinopathy screening in Rwanda. British Journal of Ophthalmology, 108(6), 840-845. https://doi.org/10.1136/bjo-2022-322683
Wong, T. Y., Sun, J., & Kawasaki, R. (2018). Guideline on Diabetic Eye Care. American Academy of Ophthalmology, 125(10), 1608-1622. https://doi.org/10.1016/j.ophtha.2018.04.007
World Health Organization. (2002). A simplified cataract grading system. Retrieved from https://iris.who.int/bitstream/handle/10665/67221/WHO_PBL_01.81.pdf
World Health Organization. (2019). World report on vision. Retrieved from https://www.who.int/publications/i/item/world-report-on-vision
Xu, X. H., Sun, B., Zhong, S., Wei, D. D., Hong, Z., & Dong, A. Q. (2020). Diabetic retinopathy predicts cardiovascular mortality in diabetes: a meta-analysis. BMC Cardiovascular Disorders, 20(1), Article 478. https://doi.org/10.1186/s12872-020-01763-z
Yang, H., & Garibaldi, J. M. (2015). A hybrid model for automatic identification of risk factors for heart disease. Journal of Biomedical Informatics, 58 Suppl, S171-S182. https://doi.org/10.1016/j.jbi.2015.09.006
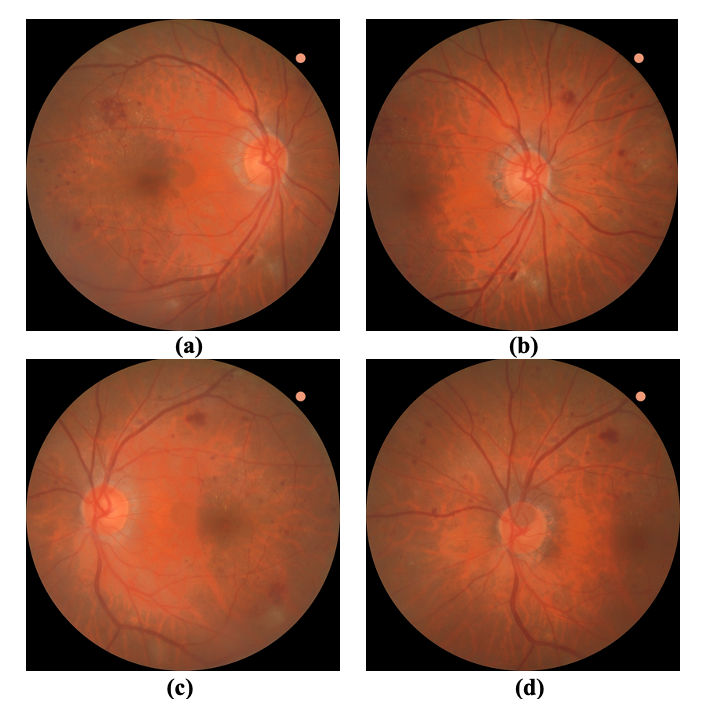
Downloads
Published
How to Cite
License
Copyright (c) 2024 Journal of Current Science and Technology

This work is licensed under a Creative Commons Attribution-NonCommercial-NoDerivatives 4.0 International License.