Prediction of Ultimate Tensile Strength of Ductile Iron by Artificial Intelligence
Abstract
Background and Objectives: In the process of ductile iron casting, predicting the mechanical properties of a cast sample from its compositions prior to pouring has been envisioned as a guideline for precision manufacturing and cost savings. Artificial intelligence holds potential for predicting the properties of ductile iron based on its chemical compositions. Thus, the present study tested the feasibility of using artificial intelligence to predict the tensile strength of ductile iron by using both multi-linear regression and multi-layer perceptron algorithms.
Methodology: In predicting the tensile strength from chemical compositions of ductile iron, a dataset comprising of six chemical compositions, i.e., carbon, silicon, manganese, sulfur, magnesium and copper, along with tensile strength data from various publications, were used. The datasets consisted of a total of 55 samples.
Main Results: The number of training datasets had an impact on the accuracy of predictions generated by both AI models. As per the results provided by the multi-linear regression algorithm, the carbon and silicon contents as well as the manganese, copper and silicon contents were significantly related to the tensile strength values. Use of the multi-layer perceptron model with appropriate parameters yielded better predictions when compared to the use of the multi-linear regression model.
Conclusions: Application of artificial intelligence to predict the tensile strength of ductile iron holds significant feasibility.
Practical Application: Based on the results, which indicate that artificial intelligence can be used to predict the tensile strength of ductile iron prior to casting, the artificial intelligence model exhibits potential to expand to the prediction of properties of ductile iron in the casting industry.
References
International ISO Standard, 2004, ISO 1083:2004E, Spheroidal Graphite Cast Irons Classification, pp. 1-7.
Palatto, L.F., Gutiếrrez, L., Vargas, E. and Bertha, L., 2020, “A Methodology for Optimization of Mechanical Properties of Automotive Iron-Casting Brakes using Artificial Neural Networks,” Procedia Manufacturing, 52, pp. 162-167.
Song, L., Zhang, H., Zhang, J. and Guo, H., 2024, “Prediction of Heavy-section Ductile Iron Fracture Toughness Based on Machine Learning,” Scientific Reports, 14 (4681). https://doi.org/10.1038/s41598-024-55089-3
Behera, R.K., Swain, S.K., Sen, S. and Mishra, S.C., 2013, “Property Prediction of Ductile Iron (DI): Artificial Neural Network Approach,” Orissa Journal of Physics, 20 (2), pp.217-224.
Çehiz, C., 2019, Cooling Curve Thermal Analysis and Oxygen Activity, Master of Natural and Applied Sciences Thesis, Middle East Technical University, 105 p.
Vitor, E.A.A., 2015, Use of Thermal Analysis to Control the Solidification Morphology of Nodular Cast Irons and Reduce Feeding Needs, Doctoral of Mechanical and Process Engineering Thesis, University of Duisburg-Essen, 182 p.
Swain, S.K., 2008, Effect of Chemistry and Processing Variables on the Mechanical Properties of Thin-wall Ductile Iron Castings, Master of Metallurgical and Materials Engineering Thesis, National Institute of Technology Rourkela, 86 p.
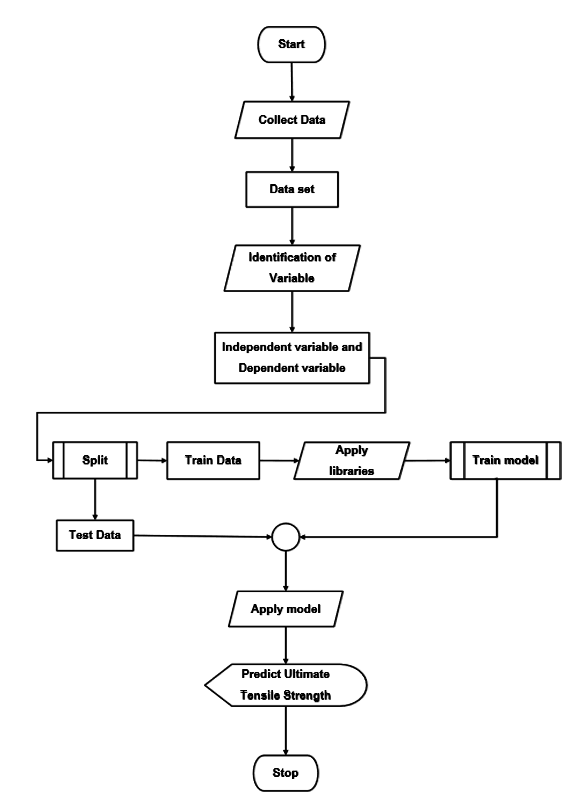
Downloads
Published
How to Cite
Issue
Section
License
Copyright (c) 2024 King Mongkut's University of Technology Thonburi

This work is licensed under a Creative Commons Attribution-NonCommercial-NoDerivatives 4.0 International License.
Any form of contents contained in an article published in Science and Engineering Connect, including text, equations, formula, tables, figures and other forms of illustrations are copyrights of King Mongkut's University of Technology Thonburi. Reproduction of these contents in any format for commercial purpose requires a prior written consent of the Editor of the Journal.